By John San Filippo
The shortcomings of the decades-old, yet still widely used, credit scoring systems are well-known. While they make short work of handling loan applicants with either good credit or bad credit, they struggle with borrowers who simply don’t fit that model – borrowers with thin credit files, no credit files or “bumpy” credit files. These borrowers are typically denied outright and forced to go to high-interest, high-risk lenders.
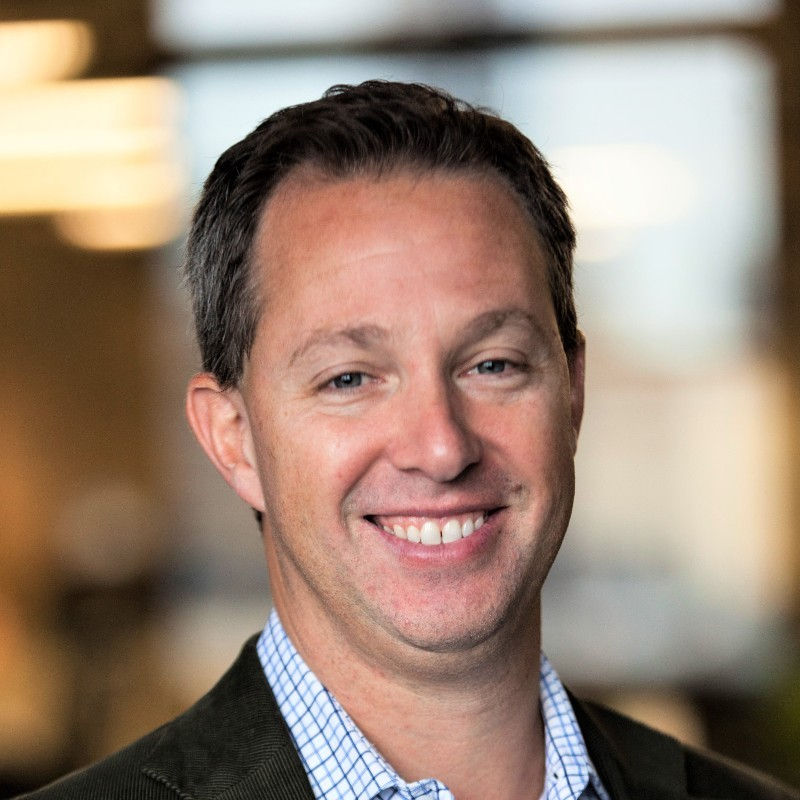
Credit unions know that some of these underserved consumers would make good borrowers. However, traditional credit scoring lacks the tools to make an accurate prediction for any given member. These institutions would gladly say “yes” if given a solid reason to do so.
One company, Edge, has developed an underwriting system that analyzes member cashflow data much like a business might have its cashflow looked at when seeking credit. In other words, by applying its technology to a member’s transactional data that’s already held by the credit union, Edge can predict credit worthiness without ever looking at the member’s credit report.
Finopotamus spoke with Edge CEO Brian Reshefsky to learn more about this technology.
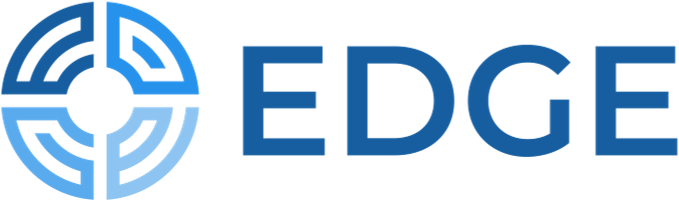
The Basics
“Edge is a cashflow bureau, so not dissimilar to a credit bureau,” Reshefsky told Finopotamus. “We give credit unions the ability to assess consumer risk using cashflow data, which is coming exclusively from that member’s transaction data with their credit union account.”
Reshefsky said that he was working for a company that used cashflow data for business analysis and evaluation when he realized the predictive power of that data. He described the technology at that time as “clunky and cumbersome,” making ill-suited for any consumer application.
Over time, the technology improved. First, the rise of data aggregators like Yodlee, Plaid and MX made banking data more accessible. “What that did is create a plumbing system of financial institutions where consumers could now use their transaction data,” said Reshefsky. He added that using this data, Edge is able to identify predictive “signals” within the transaction data and render a three-digital score not unlike a FICO or Vantage score.
Reshefsky explained that the actual analysis is not complicated. “If you were to sit around and look at transaction data on a consumer, you could solve for the probability of default given enough time,” he noted. “It's the tech that’s complicated because you have to get complex data sets, you have to get them fast, you have to be able to show compression without losing accuracy. That’s what we had to solve for and it took some time and it took quite a few mistakes along the way.”
He continued, “If you think about what's in a bank statement, you're looking at a consumer's profit and loss statement and their balance sheet at the same time. It can be quite messy in there, though. What is income and what isn’t? Do they have additional indebtedness and how do they spend their paycheck? Are they being responsible? Are there fraud signals in there that make you concerned? All those things tend to live within the bank transaction data. Now we can begin to triangulate on risk.”
Under the Hood
Finopotamus asked Reshefsky if two different members with the same income and outgo could get two different scores based on how each spent their money.
“We're not looking at categories like, if Person A spends their money at 7-Eleven and Person B spends their money on gas, he explained. “That categorization has been solved and it's proven to be less predictive. You could get different scores, though, because Person A spends all their money in three days and Person B takes two weeks. Now you're looking at the velocity of income or the velocity of spend versus where they spend.”
He continued, “Our models are built that way with intention because what you don't want to do is penalize someone for lifestyle choice or have any disparate impact. You also don't want to reward people for lifestyle choices. You want to reward people for financially responsible behavior, irrespective of how they choose to spend their money or how they make their money.”
Fighting the Fintechs
Reshefsky explained that while the Edge system can score members based on only credit union data, system accuracy is greatly enhanced when the credit union is able to aggregate data from other financial providers. “We're starting with the members, with the credit union’s data,” he said. “They're sitting on mounds and mounds of information on their members. If they're not utilizing it, what they're essentially doing is they're fighting with two hands behind their back against the fintech folks. Because the fintech folks are not depository institutions and are offering up often inferior products.”
Even though credit unions often offer superior financial products, he noted that they face other challenges bringing those products to market. “Oftentimes the credit unions either can't produce a decision fast enough with a high level of confidence, or they lack automation,” he said, adding that fintechs routinely use aggregated data to take business away from traditional financial institutions.
“How do we put credit unions on an equal footing? First, we let them harness their own data,” said Reshefsky. “That has to be done in real time – at light speed so they can compete head-to-head with anyone else. Then we're going to give them the ability to look outside their credit unions for financial information on their members. Credit unions can be as powerful as a JP Morgan Chase using data.”
“The credit unions that choose not to participate in the consumers taking control of their own financial access are going to struggle,” he added. “Most credit unions realize that, and they're in a very unique position right now to make a meaningful impact on that member journey. They have an opportunity to put themselves in a position to be competitive on a meaningful scale if they embrace this kind of technology.”